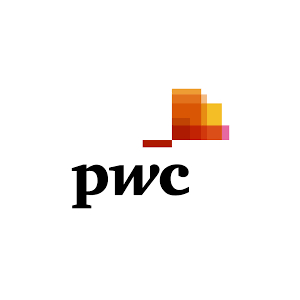
“Once again Saltmarch has knocked it out of the park with interesting speakers, engaging content and challenging ideas. No jetlag fog at all, which counts for how interesting the whole thing was."
Cybersecurity Lead, PwC
In the fecund landscape of AI, where OpenAI and Google have long been the towering trees, a new and promising bud is emerging. Meet Petals, a decentralized machine learning platform that aims to democratize the field of AI. Unlike the established flora that relies on centralized data centers and massive computational power, Petals is sowing the seeds of a decentralized approach. By leveraging peer-to-peer networks to distribute computational tasks, it aims to make machine learning accessible and affordable for everyone—from small businesses to individual researchers. This isn't just another flower in the garden; it's a potential game-changer that could redefine how we cultivate and nurture AI technologies.
Petals is an open-source initiative that has made its codebase publicly available for anyone to view, modify, and contribute to. This open-source model has been a catalyst for community involvement, allowing for rapid development and iteration. The central hub for all this collaborative action is the project's GitHub repository, where code, issues, and discussions come together in a dynamic ecosystem.
The project didn't emerge in a vacuum; it was conceived as part of the Big Science Workshop. This collaborative platform unites researchers, engineers, and data scientists from diverse fields, providing an interdisciplinary approach that has been instrumental in shaping Petals' vision and technical roadmap.
But the project extends beyond GitHub. Its official website, petals.dev, serves as a comprehensive resource for anyone interested in decentralized machine learning. The site is packed with extensive documentation that ranges from basic setup instructions to advanced use-cases. And for those who are new to the field, the website offers tutorials to help users get their feet wet.
Community support is another cornerstone of the Petals project. The team actively encourages public contributions and has established various channels for community interaction. Whether you're looking to resolve an issue, share an experience, or contribute to development, Petals offers multiple avenues through GitHub discussions and community forums.
In keeping with its commitment to openness and transparency, Petals also provides a clear roadmap of its development process. Hosted on GitHub, this roadmap outlines planned features, ongoing work, and areas where the community can contribute most effectively.
The real magic behind Petals isn't just its open-source ethos; it's the groundbreaking technology that fuels it. At its core, Petals is rewriting the rulebook on how machine learning models are built and deployed. Forget the monolithic data centers and the prohibitive costs that have long been the entry barriers to this field. Petals leverages a decentralized approach, utilizing peer-to-peer networks to distribute the computational load.
This isn't just a tweak; it's a seismic shift. Traditional machine learning models often require a centralized infrastructure, usually housed in expensive data centers with high energy costs. Petals flips this model on its head. By distributing the computational tasks across a network of peers, it democratizes access to machine learning capabilities. This is particularly transformative for small businesses and individual researchers who have been priced out of the machine learning market. Now, they can tap into this network without the need for massive upfront investment.
But don't mistake this for a simple cost-saving measure. The decentralized approach has implications that go beyond the wallet. It introduces a level of redundancy and resilience that centralized systems often lack. If one node in the network fails, the system can easily reroute tasks to other nodes, ensuring uninterrupted service. This is critical in fields like healthcare and autonomous driving, where system failures can have dire consequences.
The GitHub repository of Petals is a treasure trove for those who want to dig deeper into the tech stack. It's not just open-source; it's a living document that evolves with contributions from the community. From algorithms to network architecture, it's all laid out for those who want to get their hands dirty.
If you're not talking about Petals on social media, you're missing out on one of the most polarizing conversations in the tech community right now. This isn't your run-of-the-mill online chatter; we're diving deep into heated debates that matter to those in the know. A particular discussion recently caught fire when users began exploring the potential of building large language models through peer-to-peer training. The kicker? Petals is already ahead of the curve, pioneering this exact approach and making audacious claims about the capabilities of super-large models.
But not everyone is on the Petals bandwagon. Some have greeted the project with a healthy dose of skepticism, questioning the hype; they're probing into the very feasibility of decentralized machine learning. Can a peer-to-peer network really handle the computational demands of machine learning tasks? Is the latency too high? What about data privacy? These aren't casual critiques; they're fundamental questions that could make or break the Petals model.
What's fascinating here is the dichotomy of opinions. On one hand, you have a community that's bullish on the disruptive potential of Petals. On the other, you've got a group that's putting the project under a microscope, challenging its very foundations. This isn't just social media buzz; it's a real-time, crowd-sourced due diligence process that could shape the future of the project.
While Petals is undoubtedly a hot topic in tech circles, it's not all smooth sailing for this disruptive project. The enthusiasm is palpable, but so are the questions and doubts. Let's start with the elephant in the room: the feasibility of decentralized machine learning. This isn't just a topic for academic debate; it's a real concern that's echoed by critics who are quick to point out the challenges tied to latency and bandwidth. Can a decentralized network really deliver the speed and reliability that machine learning models require? It's a question that Petals will have to answer as it moves from concept to real-world application.
But the feasibility question isn't the only hurdle. Petals is still a fledgling project, and like any startup in its early stages, it faces the monumental task of scaling. The GitHub repository may be buzzing with activity, but can this decentralized approach handle the computational heft of larger, more complex models? It's one thing to make bold claims and generate buzz; it's another to deliver on those promises when the rubber meets the road.
What makes these challenges particularly intriguing is that they're not just technical; they're existential. If Petals can't overcome the latency and scaling issues, it risks becoming a fascinating but ultimately impractical experiment. On the flip side, if it can navigate these challenges, it has the potential to redefine the machine learning landscape entirely.
As we stroll through the AI garden, Petals stands out as a unique bloom with its audacious goals and innovative approach. It's not just a splash of color; it's a harbinger of a more democratic and cost-effective future for machine learning. But like any budding flower, it faces its own set of challenges—weather conditions, soil quality, and the ever-present skeptics who question its viability.
So, what's the final word on this intriguing bloom? It's too early for a definitive answer, but one thing is clear: Petals is a blossom worth watching. As it navigates the complex ecosystem of technological hurdles and public opinion, its resilience will be the ultimate test. Will it flourish and redefine the AI landscape, or will it wilt under the pressure? Either way, its journey promises to be as captivating as its potential impact on the garden of AI.
Have questions or comments about this article? Reach out to us here.
Banner Image Credits: Attendees at Great International Developer Summit
“Once again Saltmarch has knocked it out of the park with interesting speakers, engaging content and challenging ideas. No jetlag fog at all, which counts for how interesting the whole thing was."
Cybersecurity Lead, PwC
“Very much looking forward to next year. I will be keeping my eye out for the date so I can make sure I lock it in my calendar."
Software Engineering Specialist, Intuit
“Best conference I have ever been to with lots of insights and information on next generation technologies and those that are the need of the hour."
Software Architect, GroupOn
“Happy to meet everyone who came from near and far. Glad to know you've discovered some great lessons here, and glad you joined us for all the discoveries great and small."
Web Architect & Principal Engineer, Scott Davis
“Wonderful set of conferences, well organized, fantastic speakers, and an amazingly interactive set of audience. Thanks for having me at the events!"
Founder of Agile Developer Inc., Dr. Venkat Subramaniam
“What a buzz! The events have been instrumental in bringing the whole software community together. There has been something for everyone from developers to architects to business to vendors. Thanks everyone!"
Voltaire Yap, Global Events Manager, Oracle Corp.